The following sample references are related to our work in the Dynamic Network Lab.
Dynamic Network Theory (in order of suggested reading for newcomers)
General
Westaby, J. D., Pfaff, D. L., & Redding, N. (2014). Psychology and social networks: A dynamic network theory perspective. American Psychologist, 69, 269-284. (Good overview article of our theory - flagship journal of the APA) (Note: Our newer conceptualizing modifies system reactance (R), given the new focus on constructive reactions to conflict, such as illustrated in following Westaby and Parr, 2020, article).
Westaby, J. D., & Parr, A. K. (2020). The network goal analysis of social and organizational systems: Testing dynamic network theory in complex social networks. Journal of Applied Behavioral Science, 56(1), 107-129. Click here for copy: Network goal analysis paper (Westaby & Parr, 2020)(Good article for researchers wanting to conduct and visualize network analytics using our advanced NGA tools)
- Abstract: Grounded in dynamic network theory, this study examined network goal analysis (NGA) to understand complex systems. NGA provides new insights by inserting goal nodes into social networks. Goal nodes can also represent missions, objectives, or desires, thus having wide applicability. The theory ties social networks to goal nodes through a parsimonious set of social network role linkages, such as independent goal striving, system supporting, feedback, goal preventing, supportive resisting, and system negating (i.e., those who are upset with others in the pursuit). Moreover, we extend the theory’s system reactance role linkage to better account for constructive conflicts. Two complex systems were examined: A team’s mission and an individual’s work project. In support of dynamic network theory, using the Quadratic Assignment Procedure, results demonstrated significant shared goal striving, system supporting, and shared connections between goal striving and system supporting. These findings manifest what we coin as multipendence: Systems having some actions independently involved with goals, while others are dependently involved in the associated network. NGA also demonstrated that the goal nodes manifested strong betweenness centrality, indicating that goal striving and feedback links were connecting entities across the wider system. Strategies to plan network goal interventions are illustrated with implications for practice.
Westaby, J. D. (2012). Dynamic network theory: How social networks influence goal pursuit. Washington, DC: American Psychological Association. (The original theory - good for examining many details about DNT's multidisciplinary grounding - group researchers can also examine dedicated chapter to the topic that's not addressed in the American Psychologist article). (Note: Our newer conceptualizing modifies system reactance (R), given the new focus on constructive reactions to conflict).

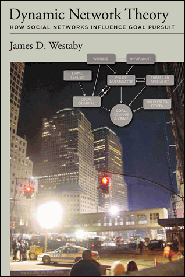
Westaby, J. D., Woods, N., & Pfaff, D. L. (2016). Extending dynamic network theory to group and social interaction analysis: Uncovering key behavioral elements, cycles, and emergent states. Organizational Psychology Review, 6, 34-62. (Good article to understand live social interactions without need for self-report survey)(Note: Our newer conceptualizing modifies system reactance (R), given the new focus on constructive reactions to conflict).
Westaby, J. D. (2020). Modeling massive social network problem solving via network goal analysis vs social network analysis. Proceedings form the Academy of Management Conference. Publication submission version in progress with Eccho Yu. (Good for examining online behavior and Big Data from a DNT perspective; no self-report surveys are needed).
Westaby, J. D., & Parr, A. K. (2018). Using network goal analysis to explain complex systems: New advances in visualization and analytics. Paper Accepted for Presentation at the North American Social Network Conference, Washington DC, November 30, 2018.
- Abstract: This research advances a powerful new platform for dynamic network analysis. Specifically, we use dynamic network theory and its network goal analysis to describe, understand, and explain how social networks influence goal achievement, performance, and climates. This study uniquely combines psychological science with social network analysis to explain important phenomena in social, organizational, and international contexts. Network goal analysis provides unique insights about system dynamics by inserting goal nodes into social networks for the first time, based on Westaby and colleagues’ theorizing. Goal nodes can also represent needs, desires, or missions at any level, thus being incredibly relevant across the human experience. This extends traditional social network analysis, which often focuses on linkages between social entities alone. Dynamic network theory ties social networks to goals through eight social network roles: goal striving (G), system supporting (S), goal preventing (P), supportive resisting (V) (e.g., indirect obstruction ties), system negating (N) (e.g., being upset with others), system reacting (R) (e.g., constructive linkages trying to resolve conflicts with others), interacting (I), and observing (O). Each of these roles are connected to the target goal or behavior under study (e.g., doing project x, getting a job, losing weight, etc.). This is also helpful because it provides a clearly bounded system for analysis. Moreover, network goal analysis and its visualization allow each sub-network to be examined separately to clearly understand behavior in complex systems. It breaks down the complexity into meaningful chunks to maximize meaning and understanding. In addition, we combine the concepts to provide overall system-level summaries of the networks, such as their positive focus ratio (G+S links / all valanced links) and network affirmation ratio (G+S+R links / divided by all valanced links). We also illustrate the power of network goal interventions to change behavior in numerous cases. A variety of other new conceptual advances grounded in dynamic network theory will also be illustrated, such as the network rippling of emotion process. This represents how emotions become contagious in networks based on goal achievement or failure. For example, "joy" would be predicted to spread throughout a network to goal strivers (G) and system supporters (S) when a goal is accomplished and communicated. In contrast, goal preventers (P) would be predicted to become more upset by the success. This illustrates the theoretical importance of having goals in social networks to explain contagion. Likewise, we demonstrate our latest conceptualizing on dynamic network intelligence (DNI), which reveals how accurate entities are in knowing other people’s social network roles for their goals. For example, Chris may think Pat is supporting Jane's goal striving, but when asked, Pat states no support for Jane's goal. Hence, Chris has low DNI on this linkage. We demonstrate how new analytics can assess this whenever data is collected on two or more people in the same goal pursuit system, such as teams. This research builds on previous work on cognitive accuracy in traditional social networks, but now contextualized to social networks involved in goal pursuit.
Lace, A., Westaby, J. D., & Coleman, P. T. (2019). Using network goal analysis to explain complex systems: New advances in visualization and analytics. Paper Accepted for Presentation at International Association for Conflict Management.
- Abstract: The purpose of this paper is to demonstrate the applications of dynamic network theory to conflict analysis and its implications for understanding complex webs of actors and planning interventions. This method and tool add value to traditional ways of analyzing conflict by incorporating goal-oriented network analysis. This argument will be made through demonstrating the application of network goal analysis to a case, provisionally selected as the current sectarian tensions in Northern Ireland in light of Brexit. The methodology involves the completion of a network goal survey by an expert. The survey assesses social network roles present in the conflict system, as well as other aspects drawn from dynamic network theory. The Qualtrics-based survey systematically explores the relationships of each entity to one another and to the goal under examination. The results are represented visually and inform the planning of interventions.
(If interested in manual graphing): Westaby, J.D. (2012):The following online resource, published with the main book above, uses old labeling from the book: G' is now P in our charts. S' is now V. R' is now N: Click here to go to the online supplemental resource A from APA. Also, it is important to note that system reactance (R) is now turned into representing constructive reactions to others if there is conflict.
Topical Applications of DNT
Westaby, J. D., & Shon, D. (2017). Simulating the Social Networks in Human Goal Striving. In R. R. Vallacher, S. J., Read, & A. Nowak (Eds.), Computational models in social psychology (1st ed.). pp. 231-257. New York, NY: Psychology Press (Frontiers of Psychology series). Recommendation: Unless you're interested in technical computional example methods, you can skip the middle section to see broader new theoretical implications (i.e., focus on the intro and latter parts of the paper).
Westaby, J. D., & Echtenkamp, A. (2017). Humor and Organizational Networks: Functions and Dysfunctions. In C. Robert (Ed.), Humor in the workplace (1st ed.). pp. 45-59. Routledge.
Westaby, J. D., & Redding, N. (2014). Social networks, social media, and conflict resolution. In P.T. Coleman, M. Deutsch, & E.C. Marcus (Eds.), The handbook of conflict resolution: Theory and practice (3rd ed.). pp. 998-1022. San Francisco, CA: Jossey-Bass.
Underlying Behavioral Intention Processes
These articles are relevant for those interested in micro behavioral intention models to predict specific behaviors. (These are not as systems-oriented):
Westaby, J. D. (2005). Behavioral Reasoning Theory: Identifying New Linkages Underlying Intentions and Behavior. Organizational Behavior and Human Decision Processes, 98, 97-120. (This is the scientific paper that introduced the theory that received considerable application across numerous behavioral contexts by external researchers).
Westaby, J. D. Probst, T. M., & Lee, B.C. (2010). Leadership Decision-Making: A Behavioral Reasoning Theory Analysis. Leadership Quarterly, 21, 481-495. This paper aimed to empirically test and validate BRT.
Wagner, M., & Westaby, J. D. (2020). Changing Pay Systems in Organizations: Using Behavioral Reasoning Theory to Understand Employee Support for Pay-for-Performance (or Not). Journal of Applied Behavioral Science, 56(3), 301-321. Click here for copy: Wagner and Westaby (2020) Winner, McGregor Best Paper Award
Westaby, J. D., Versenyi, A., & Hausmann, R. C. (2005). Intentions to Work During Terminal Illness: An Exploratory Study of Antecedent Conditions. Journal of Applied Psychology, 90, 1297-1305.
Westaby, J. D., & Lowe, J. K. (2005). Risk taking orientation and injury among youth workers: Examining the social influence of supervisors, coworkers, and parents. Journal of Applied Psychology, 90, 1297-1305.
Lee, B. C., Westaby, J. D., & Berg, D. (2004). Impact of a National Rural Youth Health and Safety Initiative: Results from a Randomized Controlled Trial. American Journal of Public Health, 94, 1743 -1749.
Order of authorship may vary depending on total writing and methodological contributions in each study. Additional author inclusions may apply.
Network goal analysis in work settings
Westaby and Parr
Multiple goal striving and feedback linkages in dynamic network theory
Parr and Westaby
Personality and network goal pursuit
Rosemarino and Westaby
New advances in behavioral reasoning theory: Predicting behavior, decision quality, and regret
Westaby and colleagues
Methodological application of network goal analysis
Parr and Westaby
The big 5 personality constructs and leadership decision making: Explaining mediational mechanisms through behavioral reasoning theory
Rosemarino and Westaby
The network goal analysis of massive online communities: A multiple goal node analysis
Westaby and Yu
Individual and group decision making: A dynamic network and behavioral reasoning approach
Mah and Westaby
Behavioral reasoning theory and remote working during the pandemic
Zlupko and Westaby
The network dynamics of unethical behavior: The Bernie Madoff case
Rosemarino and Westaby
Case study analysis of international conflict: Using network goal analysis to examine the Northern Ireland conflict
Lace, Westaby, Coleman, and DNL staff
Teacher goal striving in complex social networks: Applying network goal analysis in dynamic network theory
Hibbard, Westaby, and DNL Staff
The dynamic networks involved in coping with depression: A new network goal survey approach
Westaby, Sardana, Verdeli, and Parr
Counseling interventions among refugees: Using network goal analysis to examine outcome change
Verdeli, Sardana, Westaby, and Parr